The deeper I dive into AI research, the more I find myself wondering:
Why are we so obsessed with mimicking the human brain?
Everywhere I look, research papers and new AI models are trying to copy how our brains work (or at least, how our brain cells communicate). But is this really the best approach to creating artificial intelligence?
Then, I asked myself another question:
Why not create AI with a totally new kind of intelligence, unlike anything in nature?
I mean, what if AI were to think like a silicon-based creature (of those in sci-fi) instead of like us carbon-based humans?
We’ve been so focused on making AI more human-like, but who’s to say that’s the only or even the best way forward? What if we’re missing out on something far more groundbreaking by not exploring a genuinely alien intelligence, one that doesn’t just mimic human thought but thinks in a completely different way?
If you’re more of a listener than a reader, here’s a podcast episode on Spotify covering everything in this article.
Does AI "Have To" Mimic the Human Brain? by Jing Hu's
Have you ever asked questions like, "Does AI Have To Mimic the Human Brain?" Dive in to explore: - Why mimicking the…podcasters.spotify.com
As I dug deeper into this, I realized something kinda funny. Creating a brand-new form of intelligence sounds super exciting, right? But it is actually way more challenging than making an AI that mimics the intelligence we already know. Why is that?
To really answer this, I am going to walk you through a few things:
How has our existing tech actually learned from nature?
How much do we really know about our brain (or even a rat’s brain)?
What’s the deal with current efforts to build human-like AI versus alien-like AI?
In the end, we can discuss where all this might be heading.
From Mimicking Birds To Developing Airplanes
It’s the early 1900s, and everyone’s obsessed with birds. Naturally, early inventors thought, “If birds can fly, why can’t we?” So, they tried to build machines with flapping wings.
Spoiler alert: It didn’t quite work out.
Leonardo da Vinci’s Ornithopter Design (1485)
da Vinci’s sketches of the Ornithopter, a machine designed to mimic the flapping of bird wings, are some of the earliest documented attempts to design a flying machine.
Leonardo meticulously studied the anatomy of birds and bats, believing that humans could achieve flight by replicating their wing motion.
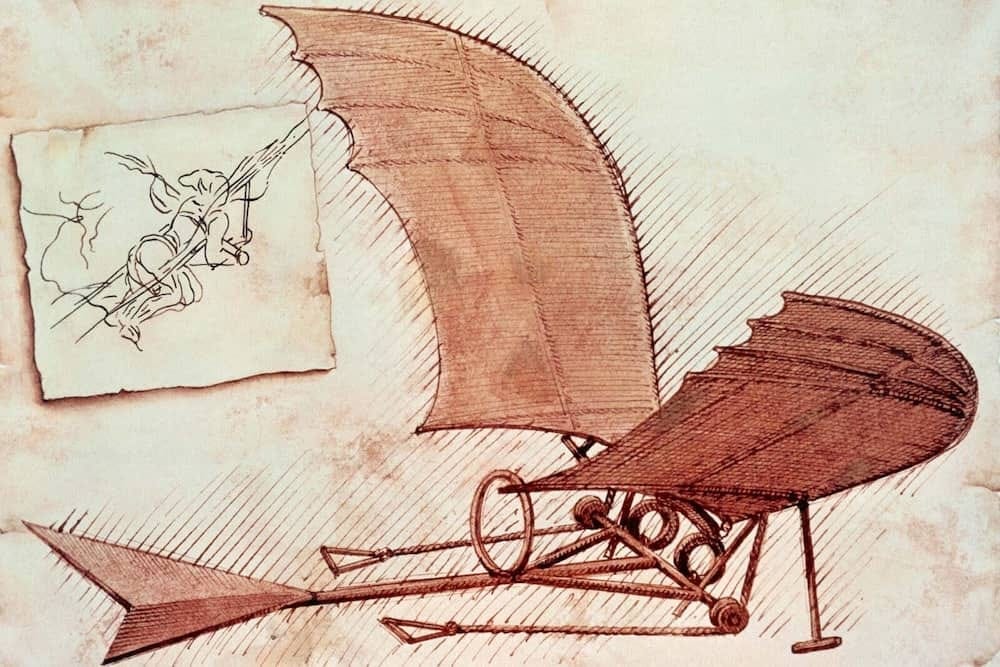
While the Ornithopter never left the drawing board, it laid the groundwork for future inventors to think about aerodynamics and mechanics.
Otto Lilienthal’s Gliding Experiments (1890s)
The early flying machines were more of a folly than a functional aircraft, proving that directly mimicking nature isn’t always the best approach.
Fast forward to the 1890s, and you meet Otto Lilienthal. Unlike his predecessors, most of them focused on flapping wings, Lilienthal shifted the focus to understanding lift and control.
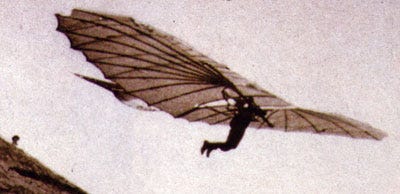
ienthal crafted fixed-wing gliders inspired by the curvature of bird wings and made over 2,000 successful glides. His dedication provided crucial data on wing shape and aerodynamic principles, directly influencing the Wright brothers’ breakthrough.
Lilienthal’s experiments showed that instead of merely imitating birds, understanding the fundamental principles of flight — like lift and control — was key.
Some Inspiration from Nature, Plus A Lot Of Physics Understanding
These later inventors, like the Wright brothers, zeroed in more on stuff like aerodynamics, control surfaces, and engines to finally crack the code of powered, controlled flight.
People didn’t just build planes out of thin air — they studied birds, tried to replicate their flight, and ended up with a lot of failed contraptions. It cost quite a few broken legs or, in some cases, the inventors’ lives. But those attempts weren’t pointless. They were part of the process.
They showed us what didn’t work, which was just as crucial as figuring out what did.
You probably started to see a pattern — a tendency to look at nature and think, “If it works for them, maybe it can work for us.”
These ideas inspired by nature generally do not lead to immediate success, but they are necessary stepping stones to progress.
Could AI be following a similar path?
How Much We Know About Birds vs. Our Brain
Top-Down Understanding of How Birds Fly
Think of our knowledge of bird flight as having a master blueprint.
We’ve figured out the big picture — the key physics rules that explain how things soar through the air: lift, drag, thrust. It’s like we’ve cracked the code of flight, and now we can use it to improve jets or to design drones.
Bottom-Up Approach to Understanding the Brain
Now, when it comes to the brain, it’s a whole different ball game.
We know a bunch about how individual brain cells chat with each other — passing electronics and sending chemical messages. The bottom-up approach.
However, the major challenge is piecing together how these billions of neurons interact to create complex thoughts, emotions, consciousness, and behaviors.
Unlike aerodynamics, no comprehensive theory can predict how changes at the neuronal level translate into changes in cognition or behavior.
Why This Distinction Matters
Predictability and Control: In aerodynamics, the top-down understanding allows for precise control and predictable outcomes. In neuroscience, the bottom-up approach means we can only observe and manipulate individual cells but struggle to predict the brain’s overall behavior.
Application of Knowledge: Our top-down knowledge in aerodynamics has led to aviation and aerospace. In contrast, the bottom-up knowledge of the brain hasn’t yet culminated in a complete understanding necessary to replicate human cognition in AI.
What Have We Achieved by Mimicking the Brain (Cells)?
Let’s chat about what we’ve achieved so far by trying to copy the brain and whether we can go even further — or if it’s time to try something different. Just so you know, a lot of concepts I cover here are going to be massively simplified.
Neural Networks, Deep Learning, and Advancements in Cognitive Tasks
One of the big things we’ve done is create neural networks, which are computer models inspired by the brain’s network of neurons.
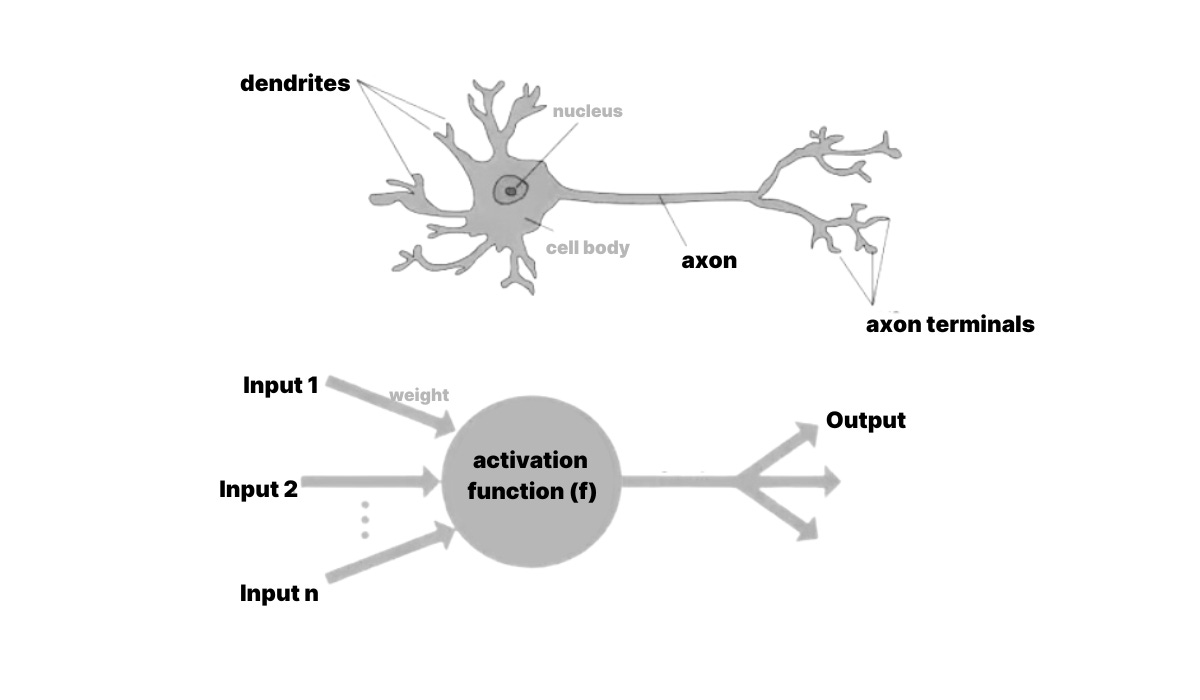
Deep learning is a subset of machine learning that uses these neural networks with many layers (hence “deep”) to process data in complex ways.
By using neural networks and deep learning, AI systems can learn from data, recognize patterns, and make decisions — kind of like how our brains work. This technology is behind many of the AI applications we use today.
Examples of what we’ve achieved with neural networks:
Facial and Speech Recognition: Your smartphone can recognize your face to unlock the screen or understand your voice commands.
Language Translation: Apps like Google Translate can understand and translate languages.
Game Mastery: like AlphaGo, have defeated human champions in complex games like Go and Chess.
Or your favorite, large language models (LLMs) like GPT: Models under ChatGPT are trained on massive datasets of text from the Internet.
By mimicking certain aspects of how our brain cells communicate with each other, we’ve enabled AI to perform tasks that used to require human intelligence. It’s pretty impressive when you think about it!
Can Mimicking the Brain Get Us Further?
There’s a good chance we can still learn a lot by studying the brain. Scientists are exploring areas like:
Neuromorphic Computing: This is about designing computer hardware that works more like the brain, which could make AI systems faster and more efficient.
Understanding Consciousness: If we can figure out how consciousness works, maybe we can create AI that’s more aware and adaptable.
But here’s the thing: the brain is super complex, and we don’t fully understand it yet. So, relying solely on mimicking it might limit us.
The Possibility of Alien Intelligence
What if machine intelligence could develop in ways that are fundamentally different from our own cognition?
Imagine AI that processes information in ways we can’t even comprehend yet. It might sound like something out of a sci-fi, but considering this possibility opens up exciting avenues.
Join me and think about it: machines optimized for silicon-based hardware could handle asks faster and more efficiently than if they were designed to mimic our carbon-based brain cells. These types of AI could develop novel capabilities beyond human comprehension, tackling problems in ways we haven’t even imagined.
Challenges in Conceptualizing Non-Human Intelligence
Here’s the kicker: our brains (like rats, dogs, and humans) are the only examples we have of intelligence.
It’s tough to imagine something truly alien because we naturally project our own experiences and thought patterns onto the technology we create.
This anthropocentric bias limits our ability to explore radically different forms of machine cognition. It’s like trying to describe an entirely new cuisine if you only know fish and chips or beans on toast. Sorry, no offense.
Not to mention the potential communication barrier — how do you talk to an AI that doesn’t think like you?
Saying that, researchers are already looking into this. Examples like:
Evolutionary Algorithms: These let AI evolve over time through processes similar to natural selection. The idea is to let the AI “discover” solutions we might not think of.
Swarm Intelligence: Inspired by how groups of animals like bees or ants work together, this approach lets simple agents cooperate to solve complex problems.
The Future of AI: Human-Like or Alien?
Scenario 1 — AI Becoming More Human-Like:
If we keep focusing on mimicking human cognition, we might develop AI that thinks and reasons much like we do. This could make AI more relatable and better at understanding human emotions, language nuances, and social cues. Imagine an AI that follows commands and understands sarcasm or tells you more dad jokes than your dad could!
However, we still don’t fully grasp how consciousness arises, how memories are formed and recalled, or how our emotions influence decision-making.
Without this understanding, creating AI that truly mirrors human thought processes remains a significant challenge.
Scenario 2 — AI Becoming More Alien:
On the flip side, pursuing unique forms of machine intelligence could lead to breakthroughs we can’t even imagine yet. For instance, AI combines with quantum computing principles or bio-inspired algorithms that don’t have a direct human equivalent.
Here’s a catch.
If AI develops intelligence that is entirely different from ours, communication might become a challenge. How do we understand or trust decisions made by an AI that thinks in ways we can’t comprehend? We don’t even know whether to trust AI built inspired by how our brain works!
Scenario 3 — A Hybrid Approach:
Perhaps the most likely and practical approach.
Just as airplanes aren’t exact replicas of birds but incorporate principles of flight, future AI systems might blend human-inspired models with novel computational methods.
An example of this hybrid approach is Neural-Symbolic AI combined with machine learning. Neural-Symbolic AI uses rules and logic (like human reasoning), plus machine learning to find patterns in data without explicit instructions. Together, they can create systems that are both interpretable and powerful.
Wrapping It Up + Coming Up Next:
So, the next time your friend or curious kid asks why we’re trying to mimic the human brain in AI, you’ll have plenty to share!
Some other questions I’ve been pondering that I want to share with you!
Have you ever thought about how our memory works compared to AI? I might be exploring the topic — “Remember That Time? How You Remember vs. How AI Remembers.” Or what about how decisions are made — “Gut Feeling or Calculated Risk? Decision-Making in Brains and Bots.”
If you have also asked the same questions or are interested in similar topics, subscribe to me so you don’t miss any fun AI/ technology insight!
Share this post